Flann 代表近似最近邻的快速库。它实际上用于系统根据数据集选择最佳算法和最佳参数。 FlannBasedMatcher 还用于匹配或搜索一幅图像与另一幅图像的特征。该函数在 OpenCV 库中可用。它用最近邻居接近并且通常跑得比BruteForceMatcher对于各种数据集。它也适用于大型数据集。这里,特征说明符搜索最接近的数值以进行特征匹配,并且其邻居的距离计算很容易且快速完成。对于FlannBasedMatcher,我们需要传递index_params 字典和搜索参数。
该库使用一组技术来实现其速度,包括近似k-d树和随机kd-trees。这些数据结构用于组织数据,使得与其他传统方法相比可以更快地搜索最近邻居。一般来说,FLANN 的工作原理是首先使用支持的索引方法之一构建数据集的索引。索引建立后,用户可以通过使用新点查询索引来执行nearest-neighbor搜索。该索引返回距查询点最近的邻居及其距离。随机k-d树和基于优先级的K-Means都是可在FLANN中使用的高效近似最近邻搜索算法,算法的选择取决于应用程序的具体要求。
例子:
在此示例中,使用 cv2.imread() 函数加载sub-image 和主图像。然后使用 SIFT 检测器检测关键点并使用 sift.detectAndCompute() 函数计算查询和训练图像中的说明符。然后使用 cv2.FlannBasedMatcher() 类创建 FLANN 对象。 knnMatch() 函数用于执行 k-nearest 邻居搜索以查找两个最近的邻居。然后使用距离比测试过滤匹配。然后使用 cv2.drawMatches() 函数绘制良好的匹配,并使用 plt.imshow() 函数显示结果。
This is a basic example of how to use FLANN and SIFT together in OpenCV for object detection. You can also experiment with different parameters, such as the number of nearest neighbors to search for, the algorithm used in FLANN, and the threshold value used in the distance ratio test to get the best results for specific use case. for more precision you need to use different parameters according to need.
输入:
main_image:
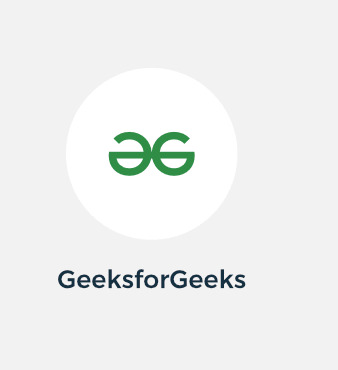
主图
sub_image:
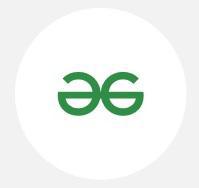
sub_image
代码说明
- Flanned_Matcher(main_image,sub_image)。
- 找到index_params并搜索参数。
- 传递给 FlannBasedMatcher(index_params,search_params) 函数。
- 匹配所有相似的特征。
- 匹配所有特征后,将其存储在另一个变量中。
- 返回存储的图像。
Python3
import cv2 as cv
import matplotlib.pyplot as plt
#Function returns the feature matched image
def Flanned_Matcher(main_image,sub_image):
# Initiating the SIFT detector
sift = cv.SIFT_create()
#Find the keypoints and descriptors with SIFT.
key_point1, descr1 = sift.detectAndCompute(main_image,None)
key_point2, descr2 = sift.detectAndCompute(sub_image,None)
# FLANN parameters.
FLANN_INDEX_KDTREE = 0
index_params = dict(algorithm = FLANN_INDEX_KDTREE, trees = 5)
search_params = dict(checks=50)
# FLANN based matcher with implementation of k nearest neighbour.
flann = cv.FlannBasedMatcher(index_params,search_params)
matches = flann.knnMatch(descr1,descr2,k=2)
# selecting only good matches.
matchesMask = [[0,0] for i in range(len(matches))]
# ratio test.
for i,(m,n) in enumerate(matches):
if( m.distance < 0.1*n.distance):
matchesMask[i]=[1,0]
draw_params = dict(matchColor = (0,255,0),
singlePointColor = (255,0,0),
matchesMask = matchesMask,flags = 0)
# drawing nearest neighbours
img = cv.drawMatchesKnn(main_image,
key_point1,
sub_image,
key_point2,
matches,
None,
**draw_params)
return img
# reading two input images
main_image = cv.imread('geeks-full.png')
sub_image = cv.imread('geeks-half.jpg')
#Passing two input images
output=Flanned_Matcher(main_image,sub_image)
# Save the image
cv2.imwrite('Match.jpg', output)
输出:
True
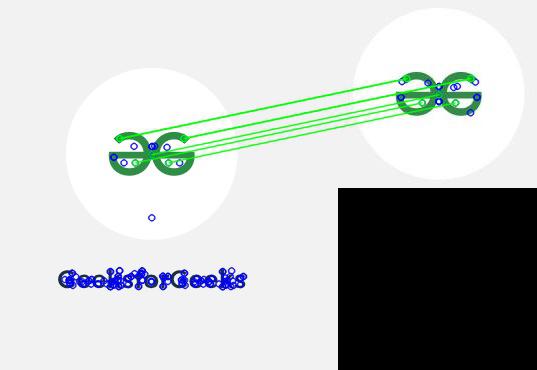
FlannBasedMatcher 输出
输出为 True 表示匹配的输出图像以名称 Match.jpg 保存在本地文件夹中
示例 2:
代码说明
- cv2.imread()是读取两个图像的图像文件。
- 使用 cv2.SIFT_create() 初始化 SIFT 检测器
- 使用 SIFT 检测器检测两幅图像中的关键点和说明符。
- 找到 index_params 并搜索 KNN 算法的参数。
- 使用 cv2.FlannBasedMatcher(index_params,search_params) 函数初始化FlannBasedMatcher。
- 匹配两个说明符之间 FlannBasedMatcher 相似的所有特征。
- 匹配所有特征后,将其存储在另一个变量中,并仅在距离小于 0.5 时过滤良好匹配(这里我们根据要求进行更改)
- 使用 cv2.drawMatchesKnn() 绘制两个图像之间的匹配
- 将匹配的图像保存到本地文件夹。
Python3
import numpy as np
import cv2
# load the images
image1 = cv2.imread('Bhagavad-Gita.jpg')
image2 = cv2.imread('Geeta.jpg')
#img1 = cv2.cvtColor(img1, cv2.COLOR_BGR2GRAY)
#img2 = cv2.cvtColor(img2, cv2.COLOR_BGR2GRAY)
# Initiate SIFT detector
sift = cv2.SIFT_create()
# find the keypoints and descriptors with SIFT
keypoint1, descriptors1 = sift.detectAndCompute(image1, None)
keypoint2, descriptors2 = sift.detectAndCompute(image2, None)
# finding nearest match with KNN algorithm
index_params = dict(algorithm=0, trees=20)
search_params = dict(checks=150) # or pass empty dictionary
# Initialize the FlannBasedMatcher
flann = cv2.FlannBasedMatcher(index_params, search_params)
Matches = flann.knnMatch(descriptors1, descriptors2, k=2)
# Need to draw only good matches, so create a mask
good_matches = [[0, 0] for i in range(len(Matches))]
# Ratio Test
for i, (m, n) in enumerate(Matches):
if m.distance < 0.5*n.distance:
good_matches[i] = [1, 0]
# Draw the matches using drawMatchesKnn()
Matched = cv2.drawMatchesKnn(image1,
keypoint1,
image2,
keypoint2,
Matches,
outImg=None,
matchColor=(0, 155, 0),
singlePointColor=(0, 255, 255),
matchesMask=good_matches,
flags=0
)
# Displaying the image
cv2.imwrite('Match.jpg', Matched)
输出:
True
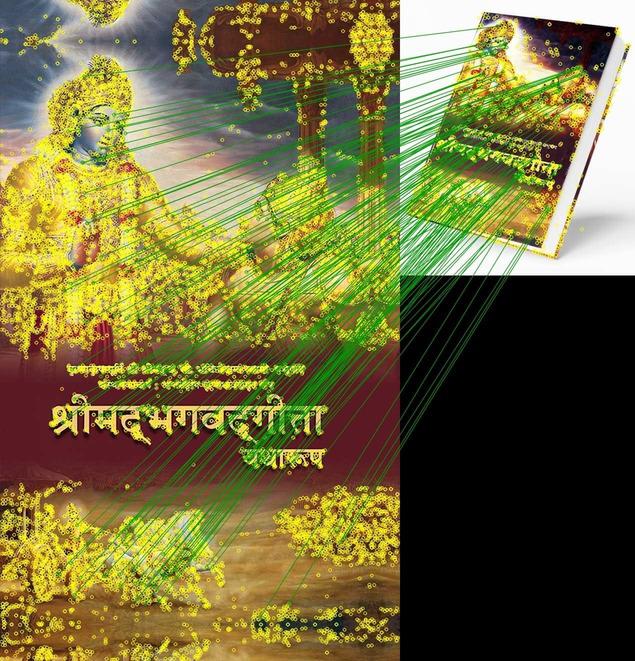
FlannBasedMatcher 输出图像
输出为 True 表示匹配的输出图像以名称 Match.jpg 保存在本地文件夹中
应用:
- 模式识别。
- 选择最佳算法和参数。
- 特征匹配。
- 数据压缩。
- 聚类分析。
- 数据库管理系统。
- 计算几何。
相关用法
- Python OpenCV Filter2D()用法及代码示例
- Python OpenCV cv2.circle()用法及代码示例
- Python OpenCV cv2.blur()用法及代码示例
- Python OpenCV cv2.ellipse()用法及代码示例
- Python OpenCV cv2.cvtColor()用法及代码示例
- Python OpenCV cv2.copyMakeBorder()用法及代码示例
- Python OpenCV cv2.imread()用法及代码示例
- Python OpenCV cv2.imshow()用法及代码示例
- Python OpenCV cv2.imwrite()用法及代码示例
- Python OpenCV cv2.putText()用法及代码示例
- Python OpenCV cv2.rectangle()用法及代码示例
- Python OpenCV cv2.arrowedLine()用法及代码示例
- Python OpenCV cv2.erode()用法及代码示例
- Python OpenCV cv2.line()用法及代码示例
- Python OpenCV cv2.flip()用法及代码示例
- Python OpenCV cv2.transpose()用法及代码示例
- Python OpenCV cv2.rotate()用法及代码示例
- Python OpenCV cv2.polylines()用法及代码示例
- Python OpenCV Canny()用法及代码示例
- Python OpenCV destroyAllWindows()用法及代码示例
- Python OpenCV getgaussiankernel()用法及代码示例
- Python OpenCV getRotationMatrix2D()用法及代码示例
- Python OpenCV getTrackbarPos()用法及代码示例
- Python OpenCV haveImageReader()用法及代码示例
- Python OpenCV imdecode()用法及代码示例
注:本文由纯净天空筛选整理自dhanushrajsrmq47大神的英文原创作品 Python OpenCV – FlannBasedMatcher() Function。非经特殊声明,原始代码版权归原作者所有,本译文未经允许或授权,请勿转载或复制。