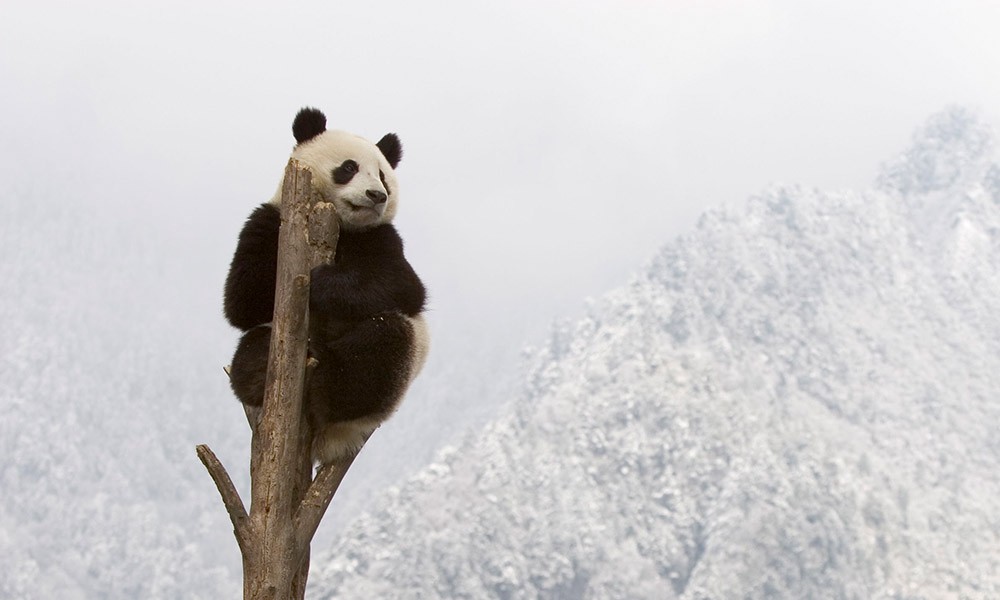
本文探討了使用Pandas DataFrame對時間序列數據的操作方法和技巧。
時間序列數據
使用給定格式將列轉換為日期時間
df[‘day_time’] = pd.to_datetime(df[‘day_time’], format=’%Y-%m-%d %H:%M:%S’)
0 2012–10–12 00:00:00
1 2012–10–12 00:30:00
2 2012–10–12 01:00:00
3 2012–10–12 01:30:00
Re-index一個DataFrame(數據幀)以內插缺失值(例如,下麵每30分鍾一次)。在運行它之前,您需要在df上有一個datetime索引。
full_idx = pd.date_range(start=df[‘day_time’].min(), end=df[‘day_time’].max(), freq=’30T’)
df = (
df
.groupby(‘LCLid’, as_index=False)
.apply(lambda group: group.reindex(full_idx, method=’nearest’))
.reset_index(level=0, drop=True)
.sort_index()
)
在DataFrame中查找缺失的日期
# Note date_range is inclusive of the end date
ref_date_range = pd.date_range(‘2012–2–5 00:00:00’, ‘2014–2–8 23:30:00’, freq=’30Min’)
ref_df = pd.DataFrame(np.random.randint(1, 20, (ref_date_range.shape[0], 1)))
ref_df.index = ref_date_range
# check for missing datetimeindex values based on reference index (with all values)
missing_dates = ref_df.index[~ref_df.index.isin(df.index)]
missing_dates
>>DatetimeIndex(['2013-09-09 23:00:00', '2013-09-09 23:30:00',
'2013-09-10 00:00:00', '2013-09-10 00:30:00'],
dtype='datetime64[ns]', freq='30T')
根據日期時間列中的日期拆分DataFrame(數據框)
split_date = pd.datetime(2014,2,2)
df_train = df.loc[df[‘day_time’] < split_date]
df_test = df.loc[df[‘day_time’] >= split_date]
在DataFrame(數據框)中找到最近的日期(這裏我們假設索引是日期時間字段)
dt = pd.to_datetime(“2016–04–23 11:00:00”)
df.index.get_loc(dt, method=“nearest”)
#get index date
idx = df.index[df.index.get_loc(dt, method='nearest')]
#row to series
s = df.iloc[df.index.get_loc(dt, method='nearest')]
計算行中日期時間之間的增量(假設索引是日期時間)
df[‘t_val’] = df.index
df[‘delta’] = (df[‘t_val’]-df[‘t_val’].shift()).fillna(0)
計算date列與給定日期之間的運行增量(例如,此處我們使用date列中的第一個日期作為我們要與之求差的日期)。
dt = pd.to_datetime(str(train_df[‘date’].iloc[0]))
dt
>>Timestamp('2016-01-10 00:00:00')
train_df['elapsed']=pd.Series(delta.seconds for delta in (train_df['date'] - dt))
#convert seconds to hours
train_df['elapsed'] = train_df['elapsed'].apply(lambda x: x/3600)
內部處理
重置索引
data
day_time
2014-02-02 0.45
2014-02-02 0.41
df.reset_index(inplace=True)
day_time data
0 2014-02-02 0.45
0 2014-02-02 0.41
#to drop it
df.reset_index(drop=True, inplace=True)
設定索引
df = df.set_index(“day_time”)
重設索引,不要保留原始索引
df = df.reset_index(drop=True)
Drop列(刪除列)
df.drop(columns=[‘col_to_drop’,'other_col_to_drop'],inplace=True)
Rename列(重命名列)
df.rename(columns={‘oldName1’: ‘newName1’, ‘oldName2’: ‘newName2’}, inplace=True)
先按column_1,然後按column_2,按升序對DataFrame(數據框)進行排序
df.sort_values(by=['column_1', 'column_2'])
#descending
df.sort_values(by='column_1', ascending=0)
選擇(Select)
根據 Pandas 列中的值從DataFrame中選擇行
超級有用的片段
df.loc[df[‘column_name’] == some_value]
df.loc[df['column_name'].isin(some_values)]
df.loc[(df['column_name'] == some_value) & df['other_column'].isin(some_values)]
從DataFrame(數據框)中選擇列
df1 = df[['a','b']]
獲取列中的唯一值
acorns = df.Acorn.unique()
#same as
acorns = df['Acorn'].unique()
按列分組,應用操作,然後將結果轉換為DataFrame(數據框)
df = df(['LCLid']).mean().reset_index()
獲列中的值最小的行
lowest_row = df.iloc[df[‘column_1’].argmin()]
按行號選擇
my_series = df.iloc[0]
my_df = df.iloc[[0]]
按列號選擇
df.iloc[:,0]
替代(Replace替換)
將數據框中的行替換為另一個具有相同索引的數據框中的行。
#for example first I created a new dataframe based on a selection
df_b = df_a.loc[df_a['machine_id'].isnull()]
#replace column with value from another column
for i in df_b.index:
df_b.at[i, 'machine_id'] = df_b.at[i, 'box_id']
#now replace rows in original dataframe
df_a.loc[df_b.index] = df_b
用行索引替換列中的值
df.loc[0:2,'col'] = 42
遍曆行
使用迭代
for index, row in df.iterrows():
print (row["type"], row["value"])
使用itertuples(速度更快)
for row in df.itertuples():
print (getattr(row, "type"), getattr(row, "value"))
如果需要修改要迭代的行,請使用apply:
def my_fn(c):
return c + 1
df['plus_one'] = df.apply(lambda row: my_fn(row['value']), axis=1)
或者,請參見以下示例:
for i in df.index:
if <something>:
df.at[i, 'ifor'] = x
else:
df.at[i, 'ifor'] = y
NaN的
用零(或某個值)替換df或列中的NaN
df.fillna(0)
df['some_column'].fillna(0, inplace=True)
統計列內的NaN數量
df[‘energy(kWh/hh)’].isna().sum()
查找具有Nan的列,這些列的列表,然後選擇具有一個或多個NaN的列:
>#which cols have nan
df.isna().any()
#list of cols with nan
df.columns[df.isna().any()].tolist()
#select cols with nan
df.loc[:, df.isna().any()]
獲取列為NaN的行
df[df['Col2'].isnull()]
數據分析
顯示DataFrame(數據框)的最後n行
df.tail(n=2)
顯示DataFrame頭的轉置。我們將len(list(df))作為數字傳遞給head以顯示所有列
df.head().T.head(len(list(df)))
>> 0 1 2 3 4
index 2012-02-05 00:00:00 2012-02-05 00:00:00 2012-02-05 00:00:00 2012-02-05 00:00:00 2012-02-05 00:00:00
LCLid MAC000006 MAC005178 MAC000066 MAC004510 MAC004882
energy(kWh/hh) 0.042 0.561 0.037 0.254 0.426
dayYear 2012 2012 2012 2012 2012
dayMonth 2 2 2 2 2
dayWeek 5 5 5 5 5
dayDay 5 5 5 5 5
dayDayofweek 6 6 6 6 6
dayDayofyear 36 36 36 36 36
字符串運算
替換列中的特定字符
df[‘bankHoliday’] = df[‘bankHoliday’].str.replace(‘?’,’’)
連接兩列
df['concat'] = df["id"].astype(str) + '-' + df["name"]
合並
在多列上合並DataFrame
df = pd.merge(X, y, on=[‘city’,’year’,’weekofyear’])
Concat /垂直附加
df = df1.append(df2, ignore_index=True) #or frames = [df1, df2, df3]
result = pd.concat(frames)
Split(分割)
將DataFrame(數據幀)分為N個大小大致相等的DataFrame
idxs=df.index.values
chunked = np.array_split(idxs, NUM_CORES)
for chunk in chunked:
part_df = df.loc[df.index.isin(chunk)]
#run some process on the part
p= Process(target=proc_chunk, args=[part_df])
jobs.append(p)
p.start()
類型轉換
更改DataFrame(數據框)中的列類型
df_test[[‘value’]] = df_test[[‘value’]].astype(int)
增加數據
添加一個空列
df["nan_column"] = np.nan
df["zero_column"] = 0
數據類型
將“ a”和“ b”列轉換為數字,將非數字強製轉換為“ NaN”
df[['a', 'b']] = df[['a', 'b']].apply(pd.to_numeric, errors='coerce')
從字典列表創建DataFrame
df = pd.DataFrame([sig_dict, id_dict, phase_dict, target_dict])
df=df.T
df.columns=[‘signal’,’id’,’phase’,’target’]
Numpy
作為連接DataFrame(數據幀)的替代方法,可以使用numpy(對於大型合並,其內存敏感度度低於pandas-useful)
a = np.array([[1, 2], [3, 4]])
b = np.array([[5, 6], [7,8]])
a, b
>(array([[1, 2],
[3, 4]]), array([[5, 6],
[7, 8]]))
c=np.concatenate((a, b), axis=1)
c
>array([[1, 2, 5, 6],
[3, 4, 7, 8]])
df = pd.DataFrame(c)
df.head()
>0 1 2 3
0 1 2 5 6
1 3 4 7 8
for i in range(10):
df = pq.read_table(path+f’df_{i}.parquet’).to_pandas()
vals = df.values
if i > 0:
#axis=1 to concat horizontally
np_vals = np.concatenate((np_vals, vals), axis=1)
else:
np_vals=vals
np.savetxt(path+f'df_np.csv', np_vals, delimiter=",")
導入/到處(Import/Export)
按列分組,然後將每個組導出到單獨的DataFrame(數據框)中:
f = lambda x: x.to_csv(“{1}.csv”.format(x.name.lower()), index=False)
df.groupby(‘LCLid’).apply(f)
#for example our original dataframe may be:
day_time LCLid energy(kWh/hh)
289 2012–02–05 00:00:00 MAC004954 0.45
289 2012–02–05 00:30:00 MAC004954 0.46
6100 2012–02–05 05:30:00 MAC000041 0.23
以Feather格式導入/導出
在這裏,我們將DataFrame(數據框)保存為Feather格式(讀回的速度非常快)。注意在使用pandas == 0.23.4保存Feather文件>〜2GB時可能會遇到問題
df.to_feather(‘df_data.feather’)
import feather as ftr
df = ftr.read_dataframe(‘df_data.feather’)
以Parquet格式導入/導出
import pyarrow.parquet as pq
df.to_parquet(“data.parquet”)
df = pq.read_table(“data.parquet”).to_pandas()
不帶索引保存
df.to_csv('file.csv', index=False)
讀入,指定新的列名
df = pd.read_csv('signals.csv', names=['phase', 'amplitude'])
datetime64日期和時間代碼
參考這裏(https://docs.scipy.org/doc/numpy/reference/arrays.datetime.html):
Code Meaning
Y year
M month
W week
D day
時間單位:
Code Meaning
h hour
m minute
s second
ms millisecond
us microsecond
ns nanosecond
ps picosecond
fs femtosecond
as attosecond