Pandas DataFrame.join(~)
將源 DataFrame 與另一個係列或 DataFrame 合並。
參數
1.other
| DataFrame
的 Series
或 DataFrame
或 list
要連接的另一個對象。
2. on
| string
或 list
| optional
要執行聯接的源 DataFrame 的列或索引級別名稱。這 index 的other
將用於連接。如果要指定要連接的非索引列other
, 采用DataFrame merge方法相反,它有一個right_on
範圍。
3. how
| string
| optional
要執行的連接類型:
值 |
說明 |
---|---|
源 DataFrame 中的所有行都將出現在生成的 DataFrame 中。這相當於 left-join 的 SQL。 | |
右側 DataFrame 中的所有行都將出現在生成的 DataFrame 中。這相當於 right-join 的 SQL。 | |
來自源和右側 DataFrame 的所有行都將出現在生成的 DataFrame 中。這相當於 outer-join 的 SQL。 | |
在源 DataFrame 中具有匹配值的所有行都將出現在生成的 DataFrame 中。這是相當於 inner-join 的 SQL。 |
默認情況下,how="left"
。
這是說明差異的經典維恩圖:
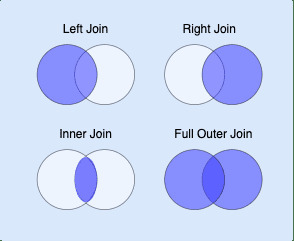
4. lsuffix
| string
| optional
附加到源 DataFrame 的重疊標簽的後綴。僅當結果中存在重複的列標簽時,這才相關。默認情況下,lsuffix=""
。
5. rsuffix
| string
| optional
附加到 other
的重疊標簽的後綴。僅當結果中存在重複的列標簽時,這才相關。默認情況下,rsuffix=""
。
6. sort
| boolean
| optional
是否根據連接鍵對行進行排序。默認情況下,sort=False
。
返回值
合並的 DataFrame
。
例子
基本用法
考慮以下關於商店的一些products
的DataFrame:
df_products = pd.DataFrame({"product": ["computer", "smartphone", "headphones"],
"bought_by": ["bob", "alex", "bob"]},
index=["A","B","C"])
df_products
product bought_by
A computer bob
B smartphone alex
C headphones bob
這是關於商店的一些customers
的DataFrame:
df_customers = pd.DataFrame({"age": [10, 20, 30]},
index=["alex","bob","cathy"])
df_customers
age
alex 10
bob 20
cathy 30
要對 df_products
的 bought_by
列執行 left-join:
df_products.join(df_customers, on="bought_by") # how="left"
product bought_by age
A computer bob 20
B smartphone alex 10
C headphones bob 20
默認情況下,other
的索引將用作連接鍵。如果您希望更靈活地選擇哪些列用於連接,請改用 merge(~)
方法。
不同連接的比較
考慮以下有關產品和客戶的DataFrames:
df_products = pd.DataFrame({"product": ["computer", "smartphone", "headphones"],
"bought_by": ["bob", "alex", "david"]},
index=["A","B","C"])
df_customers = pd.DataFrame({"age": [10, 20, 30]}, index=["alex","bob","cathy"])
[df_products] | [df_customers]
product bought_by | age
A computer bob | alex 10
B smartphone alex | bob 20
C headphones david | cathy 30
左連接
df_products.join(df_customers, on="bought_by", how="left")
product bought_by age
A computer bob 20.0
B smartphone alex 10.0
C headphones david NaN
右連接
df_products.join(df_customers, on="bought_by", how="right")
product bought_by age
B smartphone alex 10
A computer bob 20
NaN NaN cathy 30
內部聯接
df_products.join(df_customers, on="bought_by", how="inner")
product bought_by age
A computer bob 20
C headphones bob 20
B smartphone alex 10
外連接
df_products.join(df_customers, on="bought_by", how="outer")
product bought_by age
A computer bob 20
C headphones bob 20
B smartphone alex 10
NaN NaN cathy 30
指定 lsuffix 和 rsuffix
假設我們想要加入以下 DataFrame:
df_products = pd.DataFrame({"product": ["computer", "smartphone", "headphones"],
"age": [5,6,7],
"bought_by": ["bob", "alex", "bob"]},
index=["A","B","C"])
df_customers = pd.DataFrame({"age": [10, 20, 30]},
index=["alex","bob","cathy"])
product age bought_by | age
A computer 5 bob | alex 10
B smartphone 6 alex | bob 20
C headphones 7 bob | cathy 30
請注意DataFrames 都有age
列。
由於命名重疊,執行連接會產生 ValueError
:
df_products.join(df_customers, on="bought_by")
ValueError: columns overlap but no suffix specified: Index(['age'], dtype='object')
可以通過指定 lsuffix
或 rsuffix
來解決此錯誤:
df_products.join(df_customers, on="bought_by", lsuffix="_product")
product age_product bought_by age
A computer 5 bob 10
B smartphone 6 alex 10
C headphones 7 bob 20
請注意,lsuffix
和 rsuffix
僅在存在重複列標簽時才生效。
指定排序
考慮與之前相同的示例:
[df_products] | [df_customers]
product bought_by | age
A computer bob | alex 10
B smartphone alex | bob 20
C headphones bob | cathy 30
默認情況下, sort=False
,這意味著生成的 DataFrame 的行不按連接鍵 ( bought_by
) 排序:
df_products.join(df_customers, on="bought_by") # sort=False
product bought_by age
A computer bob 20
B smartphone alex 10
C headphones bob 20
設置 sort=True
會產生:
df_products.join(df_customers, on="bought_by", sort=True)
product bought_by age
B smartphone alex 10
A computer bob 20
C headphones bob 20
相關用法
- Python PySpark DataFrame join方法用法及代碼示例
- Python Pandas DataFrame empty屬性用法及代碼示例
- Python Pandas DataFrame pop方法用法及代碼示例
- Python Pandas DataFrame nsmallest方法用法及代碼示例
- Python Pandas DataFrame sample方法用法及代碼示例
- Python Pandas DataFrame items方法用法及代碼示例
- Python Pandas DataFrame max方法用法及代碼示例
- Python Pandas DataFrame swaplevel方法用法及代碼示例
- Python Pandas DataFrame agg方法用法及代碼示例
- Python Pandas DataFrame copy方法用法及代碼示例
- Python Pandas DataFrame pow方法用法及代碼示例
- Python Pandas DataFrame insert方法用法及代碼示例
- Python Pandas DataFrame lt方法用法及代碼示例
- Python Pandas DataFrame all方法用法及代碼示例
- Python Pandas DataFrame unstack方法用法及代碼示例
- Python Pandas DataFrame mean方法用法及代碼示例
- Python PySpark DataFrame filter方法用法及代碼示例
- Python Pandas DataFrame tz_convert方法用法及代碼示例
- Python Pandas DataFrame isin方法用法及代碼示例
- Python PySpark DataFrame collect方法用法及代碼示例
- Python PySpark DataFrame intersect方法用法及代碼示例
- Python PySpark DataFrame dtypes屬性用法及代碼示例
- Python Pandas DataFrame rank方法用法及代碼示例
- Python Pandas DataFrame tail方法用法及代碼示例
- Python Pandas DataFrame transform方法用法及代碼示例
注:本文由純淨天空篩選整理自Isshin Inada大神的英文原創作品 Pandas DataFrame | join method。非經特殊聲明,原始代碼版權歸原作者所有,本譯文未經允許或授權,請勿轉載或複製。