根據索引從參數軸axis
收集切片。 (不推薦使用的參數)
用法
tf.compat.v1.gather(
params, indices, validate_indices=None, name=None, axis=None, batch_dims=0
)
參數
-
params
從中收集值的Tensor
。必須至少排名axis + 1
。 -
indices
索引Tensor
。必須是以下類型之一:int32
,int64
。這些值必須在[0, params.shape[axis])
範圍內。 -
validate_indices
已棄用,什麽都不做。索引總是在 CPU 上驗證,從不在 GPU 上驗證。警告:在 CPU 上,如果發現超出範圍的索引,則會引發錯誤。在 GPU 上,如果發現超出範圍的索引,則將 0 存儲在相應的輸出值中。
-
axis
一個Tensor
。必須是以下類型之一:int32
,int64
。params
中的axis
從中收集indices
。必須大於或等於batch_dims
。默認為第一個非批量維度。支持負索引。 -
batch_dims
一個integer
。批次維度的數量。必須小於或等於rank(indices)
。 -
name
操作的名稱(可選)。
返回
-
一個
Tensor
。具有與params
相同的類型。
警告:不推薦使用某些參數:(validate_indices)
。它們將在未來的版本中被刪除。更新說明:validate_indices
參數無效。索引總是在 CPU 上驗證,從不在 GPU 上驗證。
根據 indices
從 params
軸 axis
收集切片。 indices
必須是任何維度的整數張量(通常是一維)。
Tensor.getitem
適用於標量、tf.newaxis
和 python 切片
tf.gather
擴展索引以處理索引張量。
在最簡單的情況下,它與標量索引相同:
params = tf.constant(['p0', 'p1', 'p2', 'p3', 'p4', 'p5'])
params[3].numpy()
b'p3'
tf.gather(params, 3).numpy()
b'p3'
最常見的情況是傳遞索引的單軸張量(這不能表示為 python 切片,因為索引不是連續的):
indices = [2, 0, 2, 5]
tf.gather(params, indices).numpy()
array([b'p2', b'p0', b'p2', b'p5'], dtype=object)
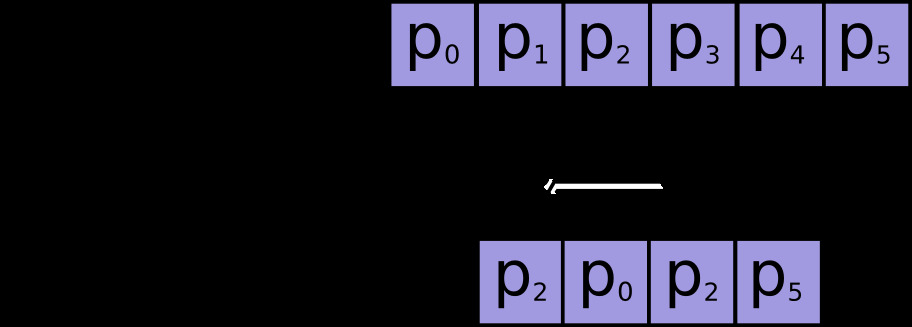
索引可以具有任何形狀。當params
有 1 個軸時,輸出形狀等於輸入形狀:
tf.gather(params, [[2, 0], [2, 5]]).numpy()
array([[b'p2', b'p0'],
[b'p2', b'p5']], dtype=object)
params
也可以具有任何形狀。 gather
可以根據 axis
參數(默認為 0)跨任何軸選擇切片。下麵它用於從矩陣中收集第一行,然後是列:
params = tf.constant([[0, 1.0, 2.0],
[10.0, 11.0, 12.0],
[20.0, 21.0, 22.0],
[30.0, 31.0, 32.0]])
tf.gather(params, indices=[3,1]).numpy()
array([[30., 31., 32.],
[10., 11., 12.]], dtype=float32)
tf.gather(params, indices=[2,1], axis=1).numpy()
array([[ 2., 1.],
[12., 11.],
[22., 21.],
[32., 31.]], dtype=float32)
更一般地說:輸出形狀與輸入形狀相同,indexed-axis 被索引的形狀替換。
def result_shape(p_shape, i_shape, axis=0):
return p_shape[:axis] + i_shape + p_shape[axis+1:]
result_shape([1, 2, 3], [], axis=1)
[1, 3]
result_shape([1, 2, 3], [7], axis=1)
[1, 7, 3]
result_shape([1, 2, 3], [7, 5], axis=1)
[1, 7, 5, 3]
這裏有些例子:
params.shape.as_list()
[4, 3]
indices = tf.constant([[0, 2]])
tf.gather(params, indices=indices, axis=0).shape.as_list()
[1, 2, 3]
tf.gather(params, indices=indices, axis=1).shape.as_list()
[4, 1, 2]
params = tf.random.normal(shape=(5, 6, 7, 8))
indices = tf.random.uniform(shape=(10, 11), maxval=7, dtype=tf.int32)
result = tf.gather(params, indices, axis=2)
result.shape.as_list()
[5, 6, 10, 11, 8]
這是因為每個索引都從 params
中獲取一個切片,並將其放置在輸出中的相應位置。對於上麵的例子
# For any location in indices
a, b = 0, 1
tf.reduce_all(
# the corresponding slice of the result
result[:,:, a, b,:] ==
# is equal to the slice of `params` along `axis` at the index.
params[:,:, indices[a, b],:]
).numpy()
True
批處理:
batch_dims
參數允許您從批次的每個元素中收集不同的項目。
使用 batch_dims=1
相當於在 params
和 indices
的第一個軸上有一個外循環:
params = tf.constant([
[0, 0, 1, 0, 2],
[3, 0, 0, 0, 4],
[0, 5, 0, 6, 0]])
indices = tf.constant([
[2, 4],
[0, 4],
[1, 3]])
tf.gather(params, indices, axis=1, batch_dims=1).numpy()
array([[1, 2],
[3, 4],
[5, 6]], dtype=int32)
這相當於:
def manually_batched_gather(params, indices, axis):
batch_dims=1
result = []
for p,i in zip(params, indices):
r = tf.gather(p, i, axis=axis-batch_dims)
result.append(r)
return tf.stack(result)
manually_batched_gather(params, indices, axis=1).numpy()
array([[1, 2],
[3, 4],
[5, 6]], dtype=int32)
batch_dims
的較高值相當於 params
和 indices
的外軸上的多個嵌套循環。所以整體形函數為
def batched_result_shape(p_shape, i_shape, axis=0, batch_dims=0):
return p_shape[:axis] + i_shape[batch_dims:] + p_shape[axis+1:]
batched_result_shape(
p_shape=params.shape.as_list(),
i_shape=indices.shape.as_list(),
axis=1,
batch_dims=1)
[3, 2]
tf.gather(params, indices, axis=1, batch_dims=1).shape.as_list()
[3, 2]
如果您需要使用諸如 tf.argsort
或 tf.math.top_k
之類的操作的索引,其中索引的最後一個維度在相應位置索引到輸入的最後一個維度,這自然會出現。在這種情況下,您可以使用 tf.gather(values, indices, batch_dims=-1)
。
也可以看看:
tf.Tensor.getitem
:直接張量索引操作(t[]
),處理標量和python-slicestensor[..., 7, 1:-1]
tf.scatter
:類似於__setitem__
(t[i] = x
)的操作集合tf.gather_nd
:類似於tf.gather
的操作,但一次收集多個軸(它可以收集矩陣的元素而不是行或列)tf.boolean_mask
,tf.where
:二進製索引。tf.slice
和tf.strided_slice
:用於對__getitem__
的 python-slice 處理(t[1:-1:2]
)的實現的較低級別訪問
相關用法
- Python tf.compat.v1.gather_nd用法及代碼示例
- Python tf.compat.v1.gfile.Copy用法及代碼示例
- Python tf.compat.v1.gfile.Exists用法及代碼示例
- Python tf.compat.v1.gradients用法及代碼示例
- Python tf.compat.v1.get_variable_scope用法及代碼示例
- Python tf.compat.v1.get_local_variable用法及代碼示例
- Python tf.compat.v1.get_variable用法及代碼示例
- Python tf.compat.v1.gfile.FastGFile.close用法及代碼示例
- Python tf.compat.v1.get_session_tensor用法及代碼示例
- Python tf.compat.v1.get_session_handle用法及代碼示例
- Python tf.compat.v1.distributions.Multinomial.stddev用法及代碼示例
- Python tf.compat.v1.distribute.MirroredStrategy.experimental_distribute_dataset用法及代碼示例
- Python tf.compat.v1.data.TFRecordDataset.interleave用法及代碼示例
- Python tf.compat.v1.distributions.Bernoulli.cross_entropy用法及代碼示例
- Python tf.compat.v1.Variable.eval用法及代碼示例
- Python tf.compat.v1.train.FtrlOptimizer.compute_gradients用法及代碼示例
- Python tf.compat.v1.layers.conv3d用法及代碼示例
- Python tf.compat.v1.strings.length用法及代碼示例
- Python tf.compat.v1.data.Dataset.snapshot用法及代碼示例
- Python tf.compat.v1.data.experimental.SqlDataset.reduce用法及代碼示例
注:本文由純淨天空篩選整理自tensorflow.org大神的英文原創作品 tf.compat.v1.gather。非經特殊聲明,原始代碼版權歸原作者所有,本譯文未經允許或授權,請勿轉載或複製。